LEGACY CONTENT.
If you are looking for Voteview.com, PLEASE CLICK HEREThis site is an archived version of Voteview.com archived from University of Georgia on
May 23, 2017. This point-in-time capture includes all files publicly linked on Voteview.com at that time. We provide access to this content as a service to ensure that past users of Voteview.com have access to historical files. This content will remain online until at least
January 1st, 2018. UCLA provides no warranty or guarantee of access to these files.
_ _
Xi Yi Xi - Xn (Xi - Xn)2 ki
---------------------------------------------------
0 -2 -2 4 -2/10 = -.200
1 -1 -1 1 -1/10 = -.100
2 1 0 0 0 = .000
3 1 1 1 1/10 = .100
4 1 2 4 2/10 = .200
---------------------------------------------------
10 0 0 10 0/10 = .000
From the Table:
åi=1,5 ki = 0
åi=1,5 kixi = (-2/10)*0+(-1/10)*1+(0)*2+(1/10)*3+(2/10)*4 = 1
_
åi=1,5 ki2 = 1/[åi=1,5 (xi - Xn)2] = 1/10 =
= (-2/10)2 + (-1/10)2 + (0)2 + (1/10)2 + (2/10)2 =
= 10/100 = 1/10 = .100
^
b1 = åi=1,5 kiyi
= (-2/10)*-2 + (-1/10)*-1 + (0)*1 + (1/10)*1 + (2/10)*1
= (4+1+0+1+2)/10 = .800
^ _ ^ _
b0 = y - b1X = 0 - (8/10)*2 = -16/10 = -1.6
- and d.
The estimated equation is:
^
yi = -1.6 + .800*xi + ei
Which produces the fitted values:
^
yi = -1.6 + .800*xi
and the estimated residuals
^ ^
ei = ei = yi - yi = yi + 1.6 - .800*xi
This produces the table
^ ^ _
xi yi yi ei = yi - yi eixi yi - y
----------------------------------------------------
0 -2 -1.6 -.400 0.0 -2
1 -1 - .8 -.200 -.2 -1
2 1 0.0 1.000 2.0 1
3 1 .8 .200 .6 1
4 1 1.6 -.600 -2.4 1
----------------------------------------------------
10 0 0.0 0.000 0.0 0
SSE = åi=1,5 ei2 = -.42 + -.22 + 12 + .22 + -.62 = 1.6
_
SSTO = åi=1,5 (yi - y)2 = -22 + -12 + 12 + 12 + 12 = 8
SSTO - SSE 8 - 1.6
R2 = ---------- = --------- = .8
SSTO 8
s2 =
[åi=1,5 ei2]/(n - 2) = 1.6/(5-2) = .53333
^ _
s2{b1} = s2/[åi=1,5 (xi - X)2] = .53333/10 = .053333
^ _
s2{b0} = [s2åi=1,5 xi2]/{n[åi=1,5 (xi - X)2]}
= (.53333*30)/(5*10) = .31999
Hence:
^
s{b1} = .23094
^
s{b0} = .56568
The hypothesis test is:
Ho: b1 = 0
H1: b1
¹ 0
Our test statistic has a t distribution with n - 2 = 3 degrees of
freedom: ^ ^
test statistic = (b1 - b1)/s{b1} = .800/.23094 = 3.4641
Using EVIEWS, P-Value (two-tail) = .04052.
Ho: b0 = 0
H1: b0
¹ 0
Our test statistic has a t distribution with n - 2 = 3 degrees of
freedom: ^ ^
test statistic = (b0 - b0)/s{b0} = -1.6/.56568 = -2.8284
Using EVIEWS, P-Value (two-tail) = .06628.
Below is the EVIEWS output. The first three columns of the table contain exactly the same values as
those calculated in parts b, f and g respectively. As for the statistics
below the table, we found R (R-squared) in part e, s (SE of regression) and
the SSE (sum of squared residuals) in part f. The
Mean of the dependent variable (Y) can be found in parts b,c or d. The 2 tail significance
values below are the same as the ones computed
above.
============================================================
LS // Dependent Variable is Y
Date: 03/02/98 Time: 22:21
Sample: 1 5
Included observations: 5
============================================================
Variable CoefficienStd. Errort-Statistic Prob.
============================================================
C -1.600000 0.565685 -2.828427 0.0663
X 0.800000 0.230940 3.464102 0.0405
============================================================
R-squared 0.800000 Mean dependent var 0.000000
Adjusted R-squared 0.733333 S.D. dependent var 1.414214
S.E. of regression 0.730297 Akaike info criter-0.339434
Sum squared resid 1.600000 Schwarz criterion -0.495659
Log likelihood -4.246107 F-statistic 12.00000
Durbin-Watson stat 1.725000 Prob(F-statistic) 0.040519
============================================================
The results of the simple linear regression are given below:
============================================================
LS // Dependent Variable is FUEL
Date: 03/02/98 Time: 22:29
Sample: 1 8
Included observations: 8
============================================================
Variable CoefficienStd. Errort-Statistic Prob.
============================================================
C 15.83786 0.801773 19.75353 0.0000
TEMP -0.127922 0.017457 -7.327679 0.0003
============================================================
R-squared 0.899489 Mean dependent var 10.21250
Adjusted R-squared 0.882737 S.D. dependent var 1.910451
S.E. of regression 0.654209 Akaike info criter-0.636340
Sum squared resid 2.567934 Schwarz criterion -0.616480
Log likelihood -6.806148 F-statistic 53.69488
Durbin-Watson stat 3.017045 Prob(F-statistic) 0.000330
============================================================
The plot looks like this:
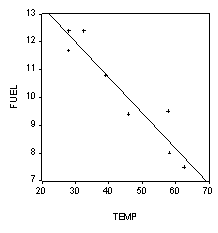
The tons of coal consumed decrease by .1279 for every degree that the
temperature decreases. At a temperature of 0, an estimate for the average
number of tons of coal consumed is 15.8378.
To find a point estimate of the expected value of fuel consumed when
the average hourly temperature is 41, we simply plug 41 into our estimated
equation: ^ ^ ^
yp = b0 + b1 xp = 15.83786 - .127922*41 = 10.593058
The 95% confidence interval for the point estimate in (c) is given by:
^ ^ _ ^
b0 + b1 xp ± t.025,n-2[s2(1 + 1/n) + (xp - X)2s2{b1}]1/2
Thus we find
15.83786 - .127922*41 ± 2.447*[.6542092(1 + 1/8) + (41 - 43.975)2(.017457)2]1/2
and the confidence limits are: (8.8904, 12.2958)
Below is the EVIEWS output for the Windmill example:
============================================================
Dependent Variable: DCOUT
Method: Least Squares
Date: 03/03/98 Time: 14:56
Sample: 1 25
Included observations: 25
============================================================
Variable CoefficientStd. Errort-Statistic Prob.
============================================================
C 0.130875 0.125989 1.038779 0.3097
WIND 0.241149 0.019049 12.65927 0.0000
============================================================
R-squared 0.874493 Mean dependent var 1.609600
Adjusted R-squared 0.869036 S.D. dependent var 0.652278
S.E. of regression 0.236052 Akaike info criter 0.027090
Sum squared resid 1.281573 Schwarz criterion 0.124600
Log likelihood 1.661381 F-statistic 160.2571
Durbin-Watson stat 0.536610 Prob(F-statistic) 0.000000
============================================================
And here is a graph of the fitted function and the residuals:
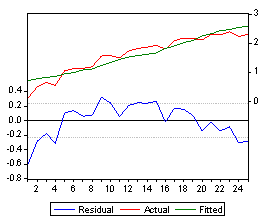
The pattern of the residuals is clearly not random. This is an
unambiguous sign that we have a specification error
and some sort of transformation of the current variables or addition of new
variables is necessary.
Below is the EVIEWS output for the paper strength example:
============================================================
Dependent Variable: TENSILE
Method: Least Squares
Date: 03/03/98 Time: 15:17
Sample: 1 19
Included observations: 19
============================================================
Variable CoefficientStd. Errort-Statistic Prob.
============================================================
C 21.32126 5.430178 3.926439 0.0011
HARDWOOD 1.770986 0.647814 2.733788 0.0141
============================================================
R-squared 0.305374 Mean dependent var 34.18421
Adjusted R-squared 0.264513 S.D. dependent var 13.77777
S.E. of regression 11.81589 Akaike info criter 7.876068
Sum squared resid 2373.458 Schwarz criterion 7.975482
Log likelihood -72.82264 F-statistic 7.473597
Durbin-Watson stat 0.246890 Prob(F-statistic) 0.014140
============================================================
And here is a graph of the fitted function and the residuals:
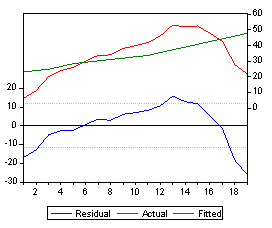
Once again the pattern of the residuals is clearly not random. This is an
unambiguous sign that we have a specification error
and some sort of transformation of the current variables or addition of new
variables is necessary.